Data Analysis
Process and Methodology
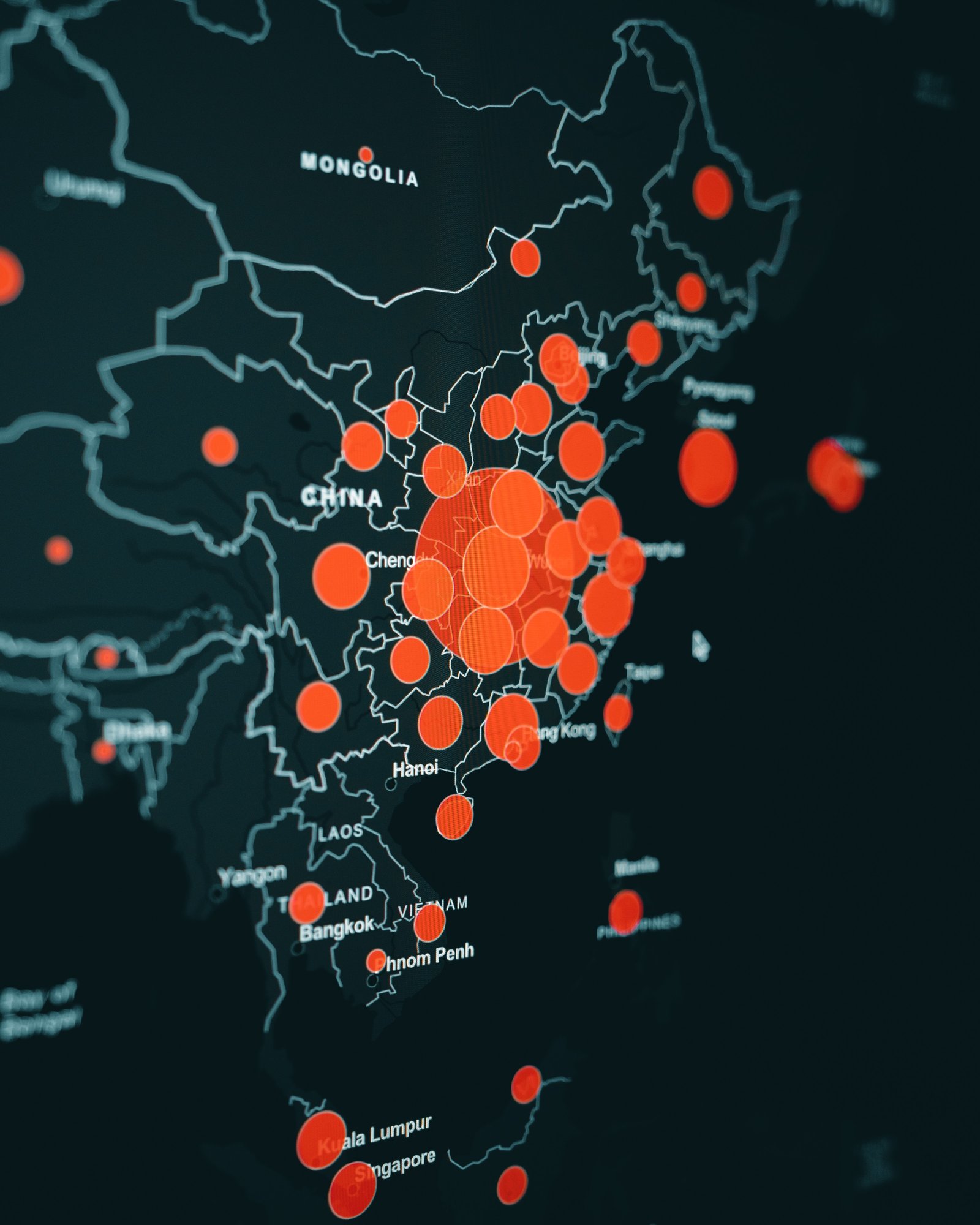
The approach
Data analysis is becoming increasingly critical in today's data-driven world, where businesses are looking to gain insights that can inform better decision-making. With the vast amount of data available, it's important to have a structured approach to data analysis that can help you make sense of it all. At Atombase, we take a comprehensive approach to data analysis, using the latest tools and techniques to help our clients gain a competitive advantage.
Our team of data analysts has a wealth of experience in working with different types of data, from customer behavior to sales data, financial data, and more. We understand that each business has its unique requirements and challenges when it comes to data analysis, and we work closely with our clients to develop customized solutions that meet their specific needs.
Our process for data analysis includes key stages such as data cleaning, data exploration, data visualization, and interpretation. By following this structured approach, we help our clients identify patterns, trends, and insights that can help them optimize performance and achieve their business objectives.
So, whether you need help with data analysis, data visualization, or data interpretation, we've got you covered. With our data analysis services, you can be confident that you are getting the insights you need to make informed decisions and stay ahead of the competition.
guidance
Navigating a strategic roadmap
Data Collection
In this stage, relevant data is identified and collected from various sources such as databases, surveys, and social media platforms. The data is then prepared and cleaned to ensure its quality and usability. Data collection also involves defining the scope of the analysis, identifying the research questions, and setting the objectives for the analysis.
Data Exploration
In this stage, the collected data is analyzed to uncover patterns, relationships, and insights. Data exploration involves the use of various techniques such as descriptive statistics, data visualization, and clustering analysis to gain a deeper understanding of the data. This stage helps in identifying any issues or errors in the data and in developing an appropriate strategy for data modeling.
Data Modeling
In this stage, statistical and machine learning algorithms are used to build predictive models that can be used to analyze the data. Data modeling involves selecting the appropriate algorithm, testing the model, and refining it based on the results. This stage also involves selecting the features that are most relevant to the analysis and developing a strategy for handling missing data.
Data Evaluation
In this stage, the model is evaluated to determine its accuracy and effectiveness. Data evaluation involves testing the model against new data sets, analyzing the results, and refining the model if necessary. This stage helps in identifying any limitations or weaknesses in the model and in developing a strategy for improvement.
Data Deployment
In this stage, the model is deployed into the production environment, and the insights generated by the analysis are used to make informed decisions. Data deployment involves developing a strategy for integrating the model into existing systems and processes and providing training to the stakeholders who will be using the model. This stage also involves developing a monitoring and evaluation plan to ensure that the model continues to perform effectively over time.
Data Maintenance
In this stage, the model is maintained and updated to ensure that it continues to perform effectively. Data maintenance involves monitoring the model's performance, updating the model as new data becomes available, and refining the model based on feedback from stakeholders. This stage also involves developing a strategy for addressing any issues or errors that may arise during the model's use.
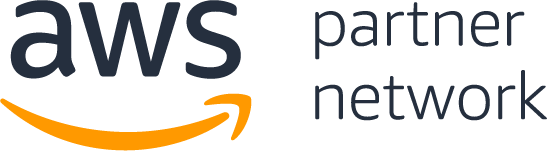
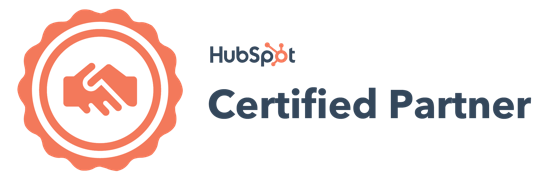
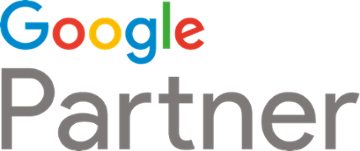